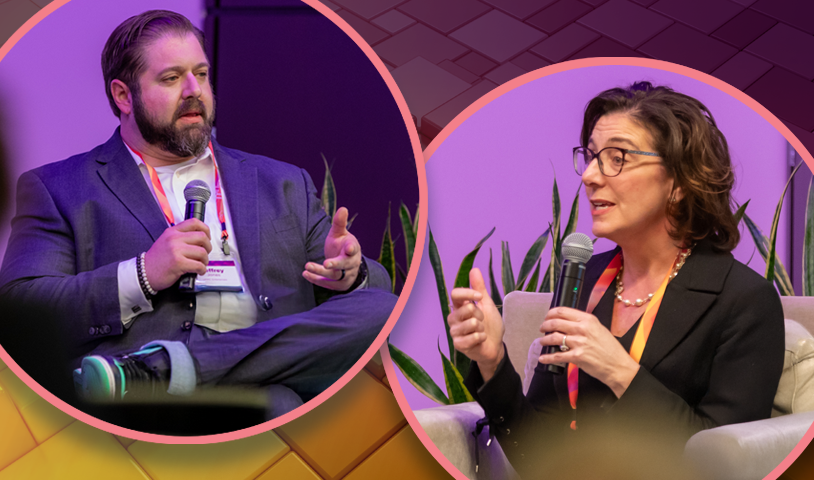
May 14, 2025
Infrastructure for Trustworthy AI: Preparing Health Systems for Responsible and Sustainable Innovation
AI is not a future consideration in health care. It is an operational reality. From diagnostics to care coordination and administrative workflows, health systems increasingly are embedding AI tools into the essential functions of care planning, delivery, and monitoring. The bigger question facing health systems and technology developers is how AI will be managed, validated, and trusted at scale.
This challenge was central to discussions at the 2025 Top of Mind Summit: Digital Health, hosted by UPMC Enterprises and the Center for Connected Medicine. While enthusiasm for AI’s potential is evident, the discussion and opinion of industry leaders focused more on one pressing issue: the need to ensure there is infrastructure to support safe, effective, and accountable AI deployment, monitoring, and maintenance.
Validation and Governance for Trustworthy AI
Health care has no shortage of AI models, but few organizations are fully prepared to implement them responsibly. Legacy IT environments, siloed data sources, and limited governance frameworks can create significant obstacles for deployment and ongoing oversight.
Speakers at the Summit emphasized that without foundational infrastructure, AI tools risk becoming unmanageable. Poorly integrated algorithms can introduce bias, erode clinician trust, and create operational inefficiencies rather than solving them.
The conversations shifted away from technical capability toward operational maturity. Success with AI will depend on whether health systems can create environments where these tools can be tested, monitored, and adapted safely over time.
A gap in many health systems when it comes to the testing and deployment of AI tools is the absence of structured validation processes. AI tools, particularly those influencing clinical decisions, require more than initial performance benchmarks. They demand continuous evaluation to track accuracy and create or sustain applicability across diverse patient populations.
Additionally, it’s highly important that clinicians and administrators have visibility or transparency into how AI models are built, how they function, and how they evolve. Without clear governance, even well-performing tools can face resistance.
The Role of Real-World Evidence
Greater availability of data along with strong infrastructure not only benefits AI deployment but also becomes a potential asset for industry partners. Regulators and payers are increasingly utilizing real-world evidence to support ongoing use of newer treatments and traditional clinical trials are insufficient for technologies that adapt over time.
This need was highlighted during the Summit by Wesley Warren, executive director of research Strategy & Partnerships at City of Hope, a leading cancer research and treatment center. Mr. Warren discussed Poseidon, City of Hope’s data infrastructure initiative designed to structure oncology data for real-world analysis. The platform supports both research and regulatory engagement by generating clinically relevant evidence directly from patient care environment.
Similarly, UPMC Enterprises recently developed and launched Ahavi™, a secure, de-identified platform for testing and validating AI models before deployment and for supporting clinical trials. These initiatives reflect a broader shift that recognizes that real-world evidence in the context of AI models is not optional for responsible AI adoption and long-term accountability.
“Partnering early with sponsors to generate real-world evidence not only shapes clinical trials but also provides insights that can drive clinician adoption. It is invaluable for both sponsors and health systems because it ensures innovation reaches the right patients without unnecessary barriers,” said Nicole Ansani, PharmD, Senior Vice President of New Development Initiatives at UPMC Enterprises.
The value of real-world evidence was echoed by Aaron Brauser, President of Realyze Intelligence, a Carta Healthcare company. “We’re partnering with pharma not only to help identify eligible patients for trials, but also to monitor outcomes after therapies are approved. Real-world data is no longer just a supplement. It’s becoming central to the entire development lifecycle,” Brauser said.
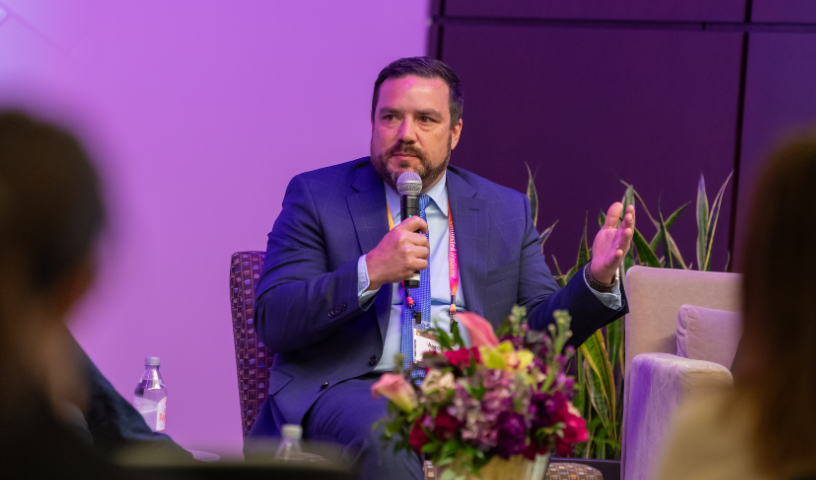
From Algorithms to Infrastructure
Jeffrey Jones, Senior Vice President of Technology Services at UPMC Enterprises, outlined a key barrier facing most health systems when it comes to the very valuable information they house, notably the fragmented data environments and the absence of controlled testing. In many health systems, legacy technology, varying data sources, and inconsistent documentation formats create challenges when trying to link data with AI models.
“Everyone talks about AI models, but few are talking about the infrastructure required to make those models usable,” Mr. Jones said. “Without clean, accessible, and well-governed data, even the most advanced algorithms struggle to function effectively within clinical workflows.”
To bridge this gap, UPMC Enterprises developed Ahavi to allow AI developers, academics, and industry to test algorithms in a controlled environment and deliver valuable insights based on real-world data. Ahavi consolidates data from across UPMC’s complex integrated delivery and financing system, allowing for comprehensive clinical and operational validation before any tool reaches patient care settings.
Unlike ad hoc testing approaches, Ahavi provides a repeatable framework where performance, bias, and reliability can be evaluated systematically. This reduces risk, accelerates deployment timelines, and ensures that AI solutions align with a system’s standards of operation and clinical care needs.
“There’s an AI tax every organization has to pay,” Mr. Jones said. “You either invest upfront in infrastructure like Ahavi, or you pay later when deployments fail or create unforeseen problems.”
Moving Beyond Pilots to Sustainable AI Operations
Another recurring insight from the Summit was the need to shift from isolated AI experiments to sustainable, scalable frameworks. Many health systems and technology partners get caught in cycles of pilot projects, lacking the infrastructure to transition successful models into enterprise-wide tools.
Standardized environments for development, testing, and monitoring can help break this cycle and allow health systems to manage AI portfolios systematically rather than through one-off efforts.
The future of AI in health care will favor organizations that treat infrastructure, governance, and validation as core competencies and not afterthoughts.
Next Steps
- Read “Seeding Innovation in Uncertain Times,” a summary of the keynote address and fireside chat featuring Dr. Robert Califf, former Commissioner of the U.S. Food and Drug Administration
- Learn more about Ahavi, our real-world data platform.
- Read all our Top of Mind reports and event coverage.